Inspecting the vast amount of infrastructure worldwide for potential faults is a time-consuming and costly process. With accelerating urban development, ensuring that buildings are safe is becoming increasingly complex and urgent.
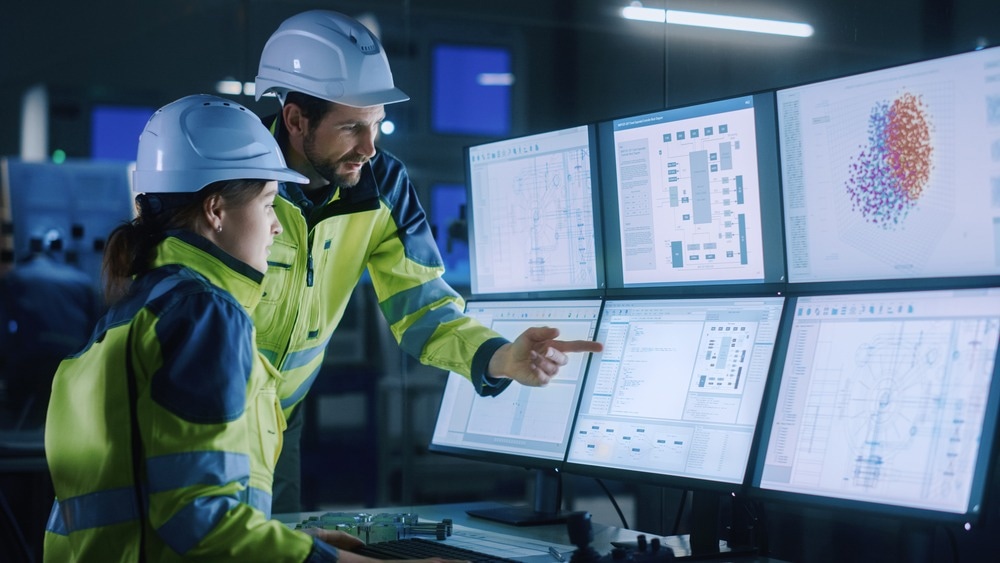
Image Credit: Gorodenkoff/Shutterstock.com
AI, robotics, and other innovative Industry 4.0 technologies offer significant benefits in terms of time, efficiency, and cost savings for infrastructure maintenance. This article explores how AI-driven robotic inspection is transforming infrastructure inspection, monitoring, and maintenance.
How is Building Inspection Commonly Performed?
Regular inspection of structures for problems such as damage, stress cracking, and subsidence is crucial to ensure their safety. This process assesses the structural integrity of buildings, protecting the lives and livelihoods of residents and communities reliant upon them.
In the construction industry, several non-destructive testing (NDT) methods are employed for this critical purpose. NDT methods do not cause any harm to materials or structural components, making them vastly preferable to destructive methods. These types of inspection methods are also cost-effective and time-efficient.
Visual inspection is one of the most predominant types of NDT inspection methods in use today, and it is also one of the oldest. However, visual inspection only gives limited information on a building’s structural soundness and cannot detect hidden defects such as corrosion, cracks, or voids.
Radiography testing uses X-Rays or gamma rays to reveal structural flaws, accurately detecting cracks, voids, and similar issues. However, interpreting the results of radiography testing is highly subjective. Eddy current testing is useful for conductive materials such as steel but cannot be applied to materials like concrete.
Ultrasonic testing is a highly effective NDT method for building inspection. It can detect poor-quality concrete, as acoustic waves travel more slowly through it than in high-quality concrete. Differences in transmission times can be interpreted to indicate the presence of structural flaws. However, in heavily reinforced concrete structures, interpreting ultrasonic testing results can be highly challenging.
Assessing the Magnitude of Infrastructure Challenges
Much of the world’s infrastructure, including bridges, dams, and tunnels, is in need of inspection and repair. According to the American Society of Civil Engineers, in the US alone, before the Infrastructure Investment and Jobs Act—a bipartisan bill signed in 2021—there was an estimated backlog of repairs needed for the nation’s bridges and roads, reaching $786 billion.
While NDT methods can provide valuable insights into the structural integrity of buildings and infrastructure, the challenges associated with standard inspection techniques in the construction industry underscore the necessity for innovative approaches that address many of the problems engineers face.
Utilizing AI-Driven Methods for Building Inspection
A multiscale robotic-driven approach to building inspection could significantly improve the efficiency of building inspection, ensuring that even minor defects do not become problematic in the future. Pre-screening structures to identify problem areas and continuously monitoring them would be an extremely powerful approach.
A research team at Drexel University has proposed an AI-driven robotic methodology for building inspection and defect monitoring. The proposed system employs precise, non-destructive laser scanning using robots, deep learning, and computer vision to detect even relatively minor faults within structures.
A high-resolution camera with stereo-depth capabilities feeds visual information to a convolutional neural network. Convolutional neural networks are currently employed in a plethora of applications across various industries, from facial recognition to detecting deepfake videos. This deep learning technology can identify even the finest discrepancies and patterns within data sets while interpreting data non-subjectively.
Convolutional neural networks and other AI-based deep learning and machine learning technologies require training on datasets. The neural networks employed in this system were trained on datasets comprising images of concrete structures and sample cracks. This allows the system to identify potential images of cracks within structures collected by the robots during laser scanning.
The neural network identifies regions of interest, which are then scanned by a robotic arm equipped with a line scanner, creating a three-dimensional digital image of the crack. At the same time, a LiDAR camera creates an image of the surrounding structure.
Both images are then stitched together to create a digital twin, which can be used to track crack growth in the structure between inspections. This provides engineers and building owners with crucial information, allowing them to better maintain the asset and repair any defects and damage before they pose a threat to life and livelihood.
Testing the System
The research team evaluated the proposed system’s performance on a concrete slab with various defects. The results of this test demonstrated the system’s high sensitivity and capability of detecting even the smallest fissures and cracks within a given structural component. Cracks less than a hundredth of a millimeter could be detected with high accuracy.
This AI-driven robotic inspection system comfortably outperforms current top-of-the-line cameras, sensors, and scanners, making it a potential building inspection tool that could overcome many of the challenges building managers and engineers face. This system would not completely negate the need for human inspectors, who would still retain the final call on maintenance.
Although this technology does not eliminate the need for human inspectors, it does reduce their workload, improving the overall performance and efficiency of routine building inspection, monitoring, and repair. It would also reduce potential oversight and mistakes caused by the subjective interpretation of datasets.
The Future
AI technologies, robotics, and automation are the future, set to revolutionize the performance of a plethora of critical tasks in multiple industries. The inspection and monitoring of the extensive global infrastructure and buildings for damage, which could lead to potentially fatal failures, stand to significantly benefit from the innovative technology developed by the research team at Drexel University.
The team intends to integrate the system into an uncrewed ground vehicle. This would create a highly efficient, comprehensive, autonomous inspection system. Real-world testing will be crucial in refining the system’s capabilities and ensuring its robustness in the construction industry. According to the research team, collaboration with regulatory bodies and industry will be essential.
Other ongoing research methods are also being explored to provide innovative solutions for the construction industry and building inspection. These include drones, augmented reality, virtual reality, and cloud-based computing approaches. The future of automated AI- and Industry 4.0-driven building inspection is, therefore, a very exciting one.
More from AZoBuild: The Use of AI in Construction
References and Further Reading
Alamdari, A.G., Ebrahimkhanlou, A. (2024). A multi-scale robotic approach for precise crack measurement in concrete structures. Automation in Construction. doi.org/10.1016/j.autcon.2023.105215
Vital Building and Pest Inspections. (2023). The Future of Building Inspections: Trends and Innovations. [Online] Vital Building and Pest Inspections. Available at: https://vitalbuildinginspection.com.au/the-future-of-building-inspections-trends-and-innovations
World Construction Today. (2019). 5 Most Common Non-Destructive Testing Methods For Building Inspection. [Online] World Construction Today. Available at: https://www.worldconstructiontoday.com/news/5-most-common-non-destructive-testing-methods-for-building-inspection/
Disclaimer: The views expressed here are those of the author expressed in their private capacity and do not necessarily represent the views of AZoM.com Limited T/A AZoNetwork the owner and operator of this website. This disclaimer forms part of the Terms and conditions of use of this website.